In 2024, generative AI has emerged as a pivotal technology, reshaping industries with innovations like ChatGPT for text generation and Midjourney for image creation. This transformation is fueled by major tech players: Microsoft’s collaboration with OpenAI and Google’s launch of Bard, an AI-driven chatbot.
Generative AI’s core function is to produce new data mimicking its training dataset, using generative models in machine learning to decode patterns in data. While various models exist for text, images, audio, codes, and videos, this rewrite focuses on video generative models.
As of 2024, the generative AI market is valued at approximately $10.8 billion, projected to $52 billion by 2028, at a CAGR of 37%.
This article explores generative model videos, highlighting their benefits and operational mechanisms. So, let’s start!
Contents
What is a Generative Video Model?
A generative video model is an advanced tool in artificial intelligence. Essentially, it’s like a digital artist that can create new videos. These models analyze tons of existing video data to understand how videos look and move.
Then, using this understanding, they generate brand new video clips that are both original and realistic. Imagine a chef tasting various dishes and then creating a unique recipe; that’s how these models work.
Unlike standard video editing software, which requires human input, generative video models do most of the work. This automation makes them incredibly useful across various fields, including movie production, video gaming, and educational content creation.
Generative Models and Their Types
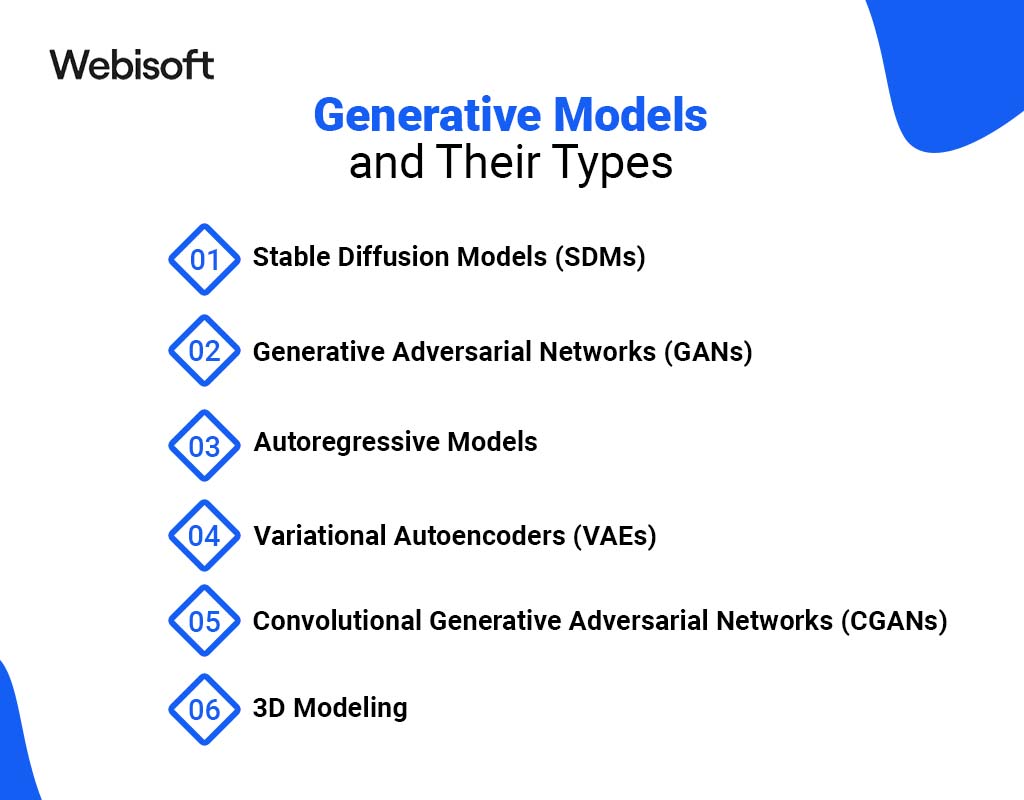
There are several generative models, each with unique capabilities and applications.
Stable Diffusion Models (SDMs)
Stable diffusion models are a newer kind of AI model. They stand out for creating highly detailed images and videos. These models are fed enormous visual data, ranging from simple drawings to complex scenes.
With this data, they learn to produce intricate and visually appealing videos. SDMs are particularly good at creating videos that have an artistic or surreal quality. They can blend different styles and concepts to create something entirely new and visually stunning.
Generative Adversarial Networks (GANs)
Generative Adversarial Networks, or GANs, involve two parts: a creator and a critic. The creator makes the video, and the critic judges it. This back-and-forth ensures the videos are realistic and high-quality.
GANs have gained popularity for their ability to produce videos that are nearly indistinguishable from real-life footage. For example, they are often used in the entertainment industry to create lifelike animations in movies and video games.
Autoregressive Models
Autoregressive models are all about predicting what comes next in a video. They look at the existing frames and then guess the future frames. This makes them excellent for creating smooth and coherent video sequences.
They are instrumental in projects where maintaining the flow and continuity of the video is essential. For instance, they can be used to create realistic animations where each frame flows seamlessly into the next.
Variational Autoencoders (VAEs)
Variational Autoencoders work by compressing and then reconstructing video data. They take detailed video information and compress it into a simpler form. Then, they use this compressed data to generate new videos.
VAEs are known for being efficient, especially with large volumes of video data. They are valuable in areas where you need to store and recreate large amounts of video content while maintaining quality.
Convolutional Generative Adversarial Networks (CGANs)
CGANs are a specialized type of GAN that focuses on understanding the layout and structure of videos. They are particularly skilled at creating videos that need to follow a specific pattern or design.
For instance, if you need to generate a video of a cityscape or a detailed interior, CGANs can create realistic and structurally accurate scenes.
3D Modeling
In 3D model video, AI-powered methods are rapidly gaining traction. These models are categorized into three subcategories based on the input format: Text-to-3D, Image-to-3D, and Video-to-3D.
Text-to-3D uses Natural Language Processing to convert textual descriptions into 3D models, Image-to-3D converts 2D images into 3D models using depth perception and object recognition, and Video-to-3D transforms video footage into 3D models or environments by analyzing movement.
While these AI-powered methods offer faster and cheaper model generation, they currently lack the quality of manual processes and have limited control over detail manipulation.
Despite these limitations, AI-generated 3D models are reshaping the industry, democratizing 3D Modeling by making it more accessible, cost-effective, and efficient for a wider audience.
What Tasks Can a Generative Video Model Perform?
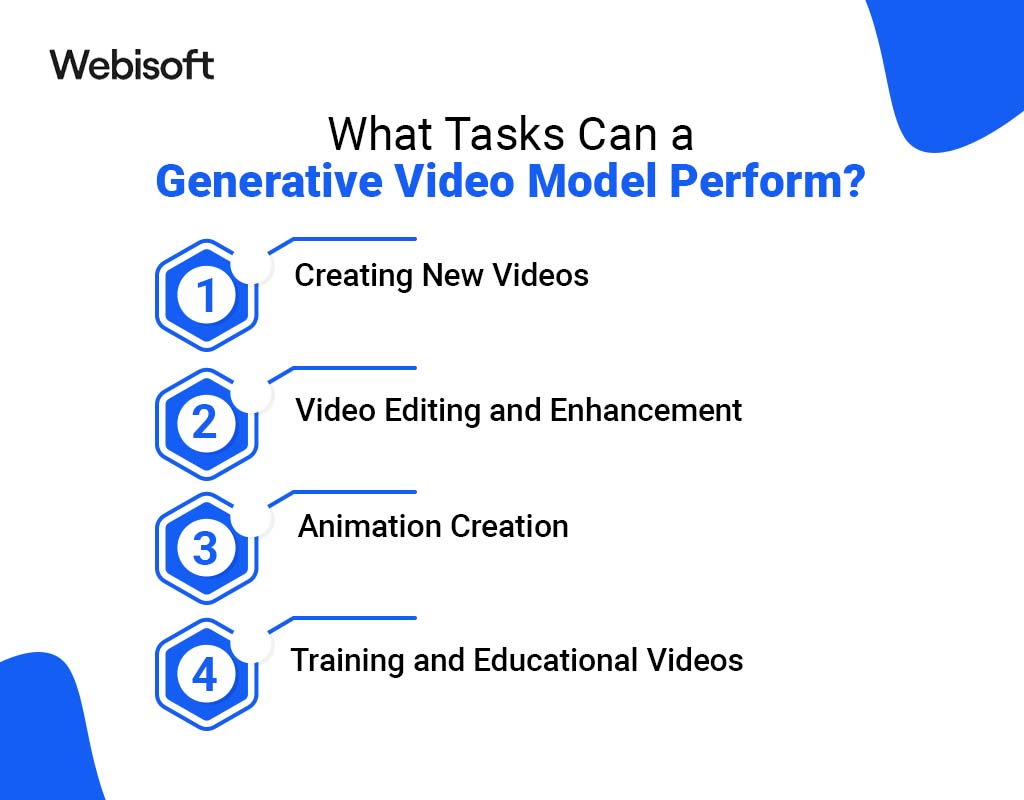
Generative video models are cutting-edge tools in the digital realm. They’re capable of various tasks that transform how we create and interact with video content.
Creating New Videos
These models are adept at generating entirely new videos. Using inputs like photos, drawings, or text descriptions, they craft visually striking and meaningful videos. This is especially useful for marketers looking to produce unique advertisements or content creators seeking fresh, original material.
Video Editing and Enhancement
Generative models can refine and enhance existing videos. They can improve resolution, adjust colors and lighting, and even add special effects or remove unwanted elements. This feature particularly benefits video editors and filmmakers striving for high-quality, polished final products.
Animation Creation
One of the standout abilities of these models is in animation. They can animate characters and scenes, making them an excellent resource for animators and graphic designers. This technology simplifies the animation process, enabling the creation of complex animated sequences with less effort.
Training and Educational Videos
In educational settings, these models play a crucial role. They can simulate real-world scenarios in a controlled, virtual environment, creating immersive and interactive training videos. This helps visualize and understand complex subjects or procedures, making it an invaluable tool for learners and trainers.
Benefits of Generative Video Models
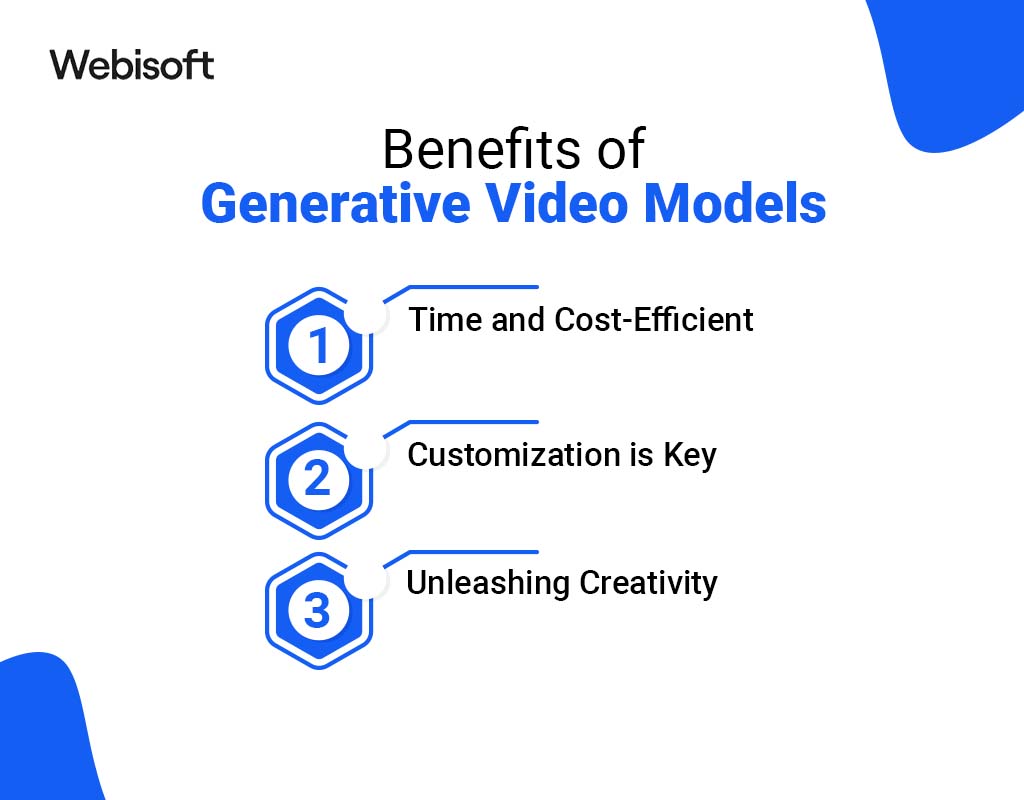
Generative video models are multifaceted tools that significantly enhance the process of video creation and editing. Their ability to save time, offer customization, boost creativity, and make high-quality video production accessible to all makes them an invaluable asset.
They’re not just shaping the present of video content creation. They’re paving the way for its future.
Time and Cost-Efficient
First and foremost, generative video models save a ton of time and money. Traditionally, creating a high-quality video could take weeks or even months and often required a hefty budget.
Now, these models streamline the process, automating many tasks that used to be manual and time-consuming. Businesses, content creators, and even hobbyists can produce top-notch videos without breaking the bank or spending endless hours on them.
Customization is Key
Another amazing benefit is the level of customization these models provide. Imagine being able to tweak every aspect of your video to match your vision or your audience’s preferences perfectly.
Whether altering the setting, changing the characters, or even adjusting the storyline, generative video models make it possible. This level of customization is a game-changer.
Unleashing Creativity
These models are a playground for creativity. They open up new possibilities that were once hard to achieve. Creators can experiment with different styles, themes, and narratives, pushing the boundaries of traditional video content. This is not just exciting for professional filmmakers and marketers.
How Do Generative Video Models Work?
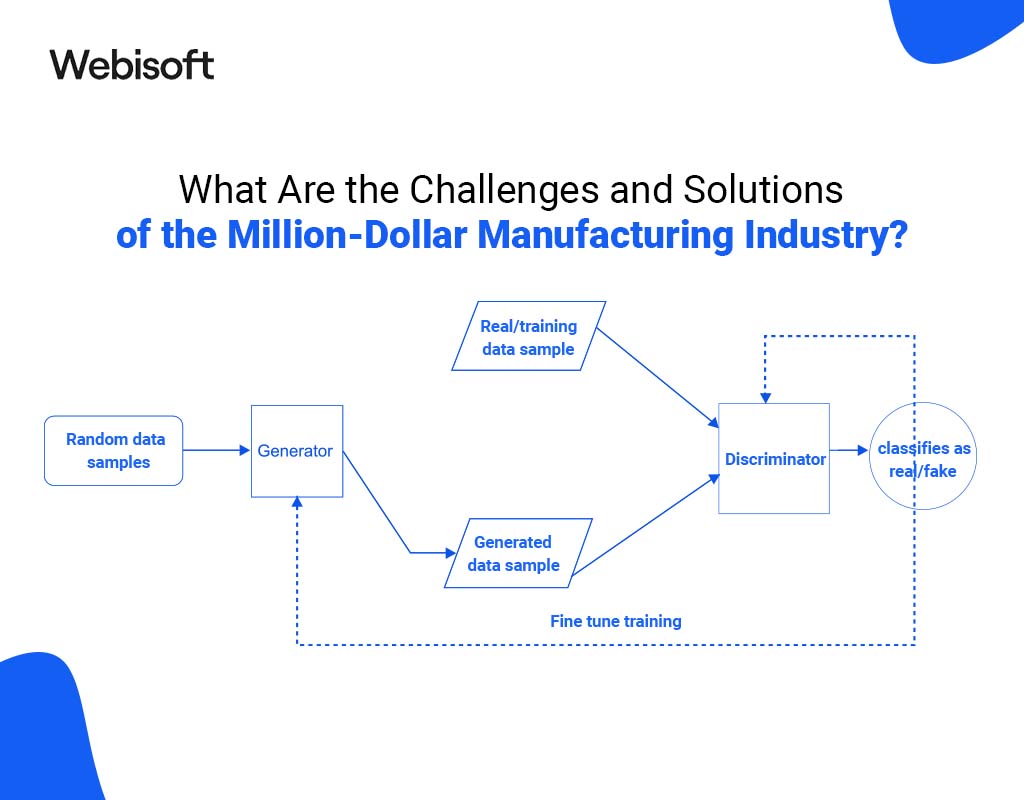
Have you ever wondered how generative video models turn simple inputs into fascinating video content? It’s a blend of art and science; understanding it can be quite intriguing. Let’s break down this process into simpler terms and explore how these models work.
The Core Concept: Machine Learning and AI
Artificial intelligence (AI), specifically a branch called machine learning, is at the heart of a generative video model.
Think of it like teaching a child to paint by showing them thousands of paintings. Eventually, the child learns to create their own artwork. Similarly, generative video models learn from a vast amount of video data.
Data: The Building Blocks
The starting point for any generative video model is lots of data. This data usually comprises a large collection of videos, images, or text, depending on what the model is intended to generate.
For instance, if the model is designed to create nature videos, it will be fed with countless hours of nature-related footage. The quality and diversity of this data significantly impact the model’s performance.
The Learning Process: Training the Model
Once the data is ready, the next step is training the model. This process involves algorithms analyzing the data identifying patterns, styles, and various elements within the videos.
The model aims to understand what makes a video appealing or relevant to a certain topic. This learning process requires powerful computer vision and can take a considerable amount of time, depending on the complexity of the task.
Generating New Content: The Creative Phase
After the model is trained, it can start generating new content. This is where it gets creative. Based on what it has learned, the model can produce new videos similar to the data it was trained on but entirely new creations.
The surprisingly sophisticated results show how well the model has learned to mimic certain styles or elements.
Refining the Output: Testing and Tweaking
The initial outputs might be flawed. That’s why testing and refining the model is crucial. By analyzing the generated videos, developers can identify areas where the model needs improvement.
They might adjust the algorithms, add more data, or tweak other parameters. This refining process is essential to enhance the quality and relevance of the videos created by the model.
Real-World Application: Bringing the Model to Life
Finally, the generative video model is ready for real-world application. It can be integration into various platforms, from social media and advertising to education and entertainment.
These models offer a new way to create efficient and engaging content, opening up endless possibilities for storytelling and visual communication.
Generative video models are a fascinating intersection of technology and creativity. They work by learning from data, training through machine learning algorithms, generating new content, and continuously refining their outputs. The potential of these models is enormous, offering new ways to create and experience video content. As technology evolves, we can only expect them to become more sophisticated and integrated into our digital experiences.
How to Create a Generative Video Model?
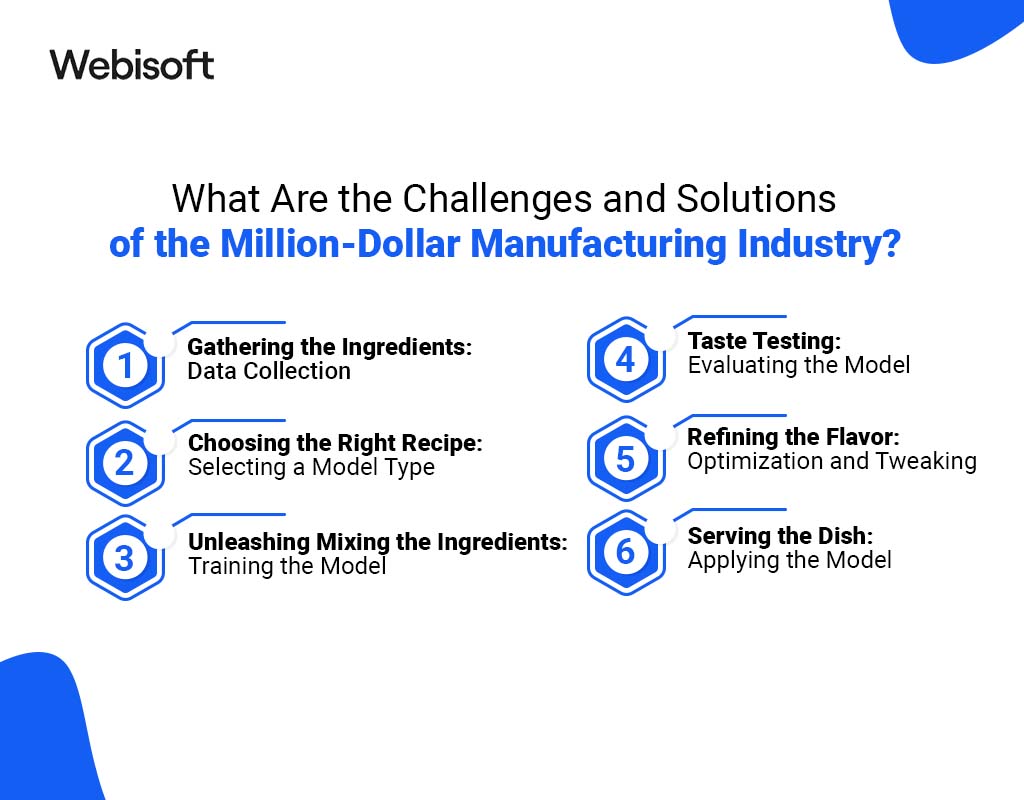
Creating a generative video model is like crafting a recipe for a new digital art. Here’s a step-by-step guide to understanding this intriguing process.
Gathering the Ingredients: Data Collection
The foundation of any generative model is the data it learns from. This means collecting a vast array of video clips for a video model. The diversity in your dataset is crucial. Include everything from animation and documentary footage to feature films and homemade videos.
Exposing the model to various styles, motions, and scenarios is the goal. Imagine teaching a language; the more words and grammar rules you know, the better you can communicate. The same goes for your model; the more diverse the data, the more versatile its video creation capabilities.
Choosing the Right Recipe: Selecting a Model Type
After gathering your data, it’s time to pick the type of generative model to train. This choice depends on your desired output. Generative Adversarial Networks (GANs) are ideal for creating highly realistic video content.
They work through a system of two neural networks – generating content and judging it. If your goal is more artistic or abstract videos, Stable Diffusion Models might be more appropriate.
These models are known for their ability to create visually stunning and unique outputs. Your choice here sets the path for the kind of videos your model will produce.
Mixing the Ingredients: Training the Model
Training the model is where the magic starts to happen. This process involves feeding the model your collected data and allowing it to learn from it. The model analyzes this data to understand color, motion, and texture.
It’s akin to a student learning from textbooks and lectures. The training process requires significant computational power and time. The model iteratively improves its understanding, refining its ability to generate new video content.
This step is critical and often the most time-consuming, but it’s where your model truly begins to develop its capabilities.
Taste Testing: Evaluating the Model
Once your model is trained, it’s time to test it. Provide it with new inputs and see how it performs. Does it generate smooth, coherent video sequences? Are the transitions between frames natural?
This evaluation is like taste-testing a dish to check if it needs more seasoning. The testing phase helps identify areas where the model might be lacking. You might find that the model struggles with certain types of video or that the quality isn’t up to par. This step is essential for understanding the strengths and weaknesses of your model.
Refining the Flavor: Optimization and Tweaking
Based on your testing results, you’ll likely need to make adjustments. This could involve retraining the model with additional data, tweaking the algorithms, or fine-tuning the parameters. Think of this as adjusting a recipe after the first taste test.
You might add more of one ingredient or cook it a bit longer. In the case of your model, you’re adjusting the ‘ingredients’ of your algorithms to improve the output quality.
Serving the Dish: Applying the Model
Now, your generative video model is ready for real-world application. You can deploy it in various domains like film production, advertising campaigns, or educational content creation.
Your model can generate unique and captivating videos, opening up new possibilities in content creation. It’s like serving a well-prepared dish to guests, ready to impress with its flavors and presentation.
Creating a generative video model is a complex process that involves careful planning, execution, and refinement. Each step, from data collection to model application, is crucial for the model’s success.
This journey not only opens up new horizons in video production but also showcases the remarkable capabilities of artificial intelligence in creative domains.
Final Note
Creating a generative model video involves a series of intricate steps, from preparing the video data to crafting the model’s structure. This process includes shaping the basic framework of the model and training it to perform well.
Often, the foundational architecture of these models is based on Generative Adversarial Networks (GANs) or Variational Autoencoders (VAEs). Layers like Convolutional, Pooling, Recurrent, or Dense are integrated to enhance the model’s abilities and intricacy.
If you’re intrigued by the potential of a generative video model and keen to harness its power for your business, consider reaching out to Webisoft. They can assist in developing a custom generative ultra model video that brings your creative vision to life.
Frequently Asked Questions
What equipment is needed to shoot a model video?
Essential equipment includes:
- A high-resolution camera.
- Lenses suitable for different shots.
- Professional lighting equipment.
- A microphone for clear audio.
- A tripod or stabilizer for steady filming.
Optional items like drones or gimbals can add dynamic shots.
How can I ensure my model video stands out?
Focus on creativity in your concept and execution. Use unique locations, innovative filming techniques, and high-quality post-production edits. Incorporate storytelling elements and ensure the model’s personality and strengths are highlighted.
What are the best platforms to share model videos?
Popular platforms include YouTube, Vimeo, Instagram, and TikTok. Choose platforms based on your audience and the video’s style. Platforms like LinkedIn or a personal portfolio website can also be effective for professional purposes.