In the ever-evolving world of technology, one term. It has been gaining significant traction in generative AI. This revolutionary technology is reshaping industries, from the creative arts to finance and everything in between.
Welcome to the fascinating realm of generative AI. A technological marvel, generative AI is making waves in various sectors.
From the creative arts to finance, its impact is far-reaching and transformative. So, what is this buzz all about? Let’s delve into the world of generative AI and unravel its mysteries.
Generative AI, artificial intelligence’s subset, is gaining prominence. Its unique ability to create new data similar to the one it’s trained on sets it apart. Imagine giving a machine the power to create – that’s generative AI models for you.
Whether it’s an image, a piece of music, or a block of text, generative AI can produce it all. The journey of generative AI is an intriguing one. Its roots trace back to the 1940s and 1950s, the era when the foundation for artificial intelligence was laid.
However, the real breakthrough came in the 2010s. Deep learning and neural networks’ advent gave generative AI the shape we recognize today.
Dive into the world of generative AI with Webisoft. Unleash your creativity, automate designs, and transform your ideas into reality.
But what exactly is generative AI, and how does it work? This comprehensive guide of Webisoft will walk you through the basics, the tech stack, different types of models, applications, and the future of generative AI.
Contents
- 1 What Is Generative AI?
- 2 A Brief History of Generative AI
- 3 The Generative AI Tech Stack
- 4 Different Types of Generative AI Models
- 5 How Does Generative AI Work?
- 6 Natural Language’s Role Processing in Generative AI
- 7 Applications of Generative AI
- 8 Generative AI Use Cases
- 9 The Future of Generative AI
- 10 End Note
What Is Generative AI?
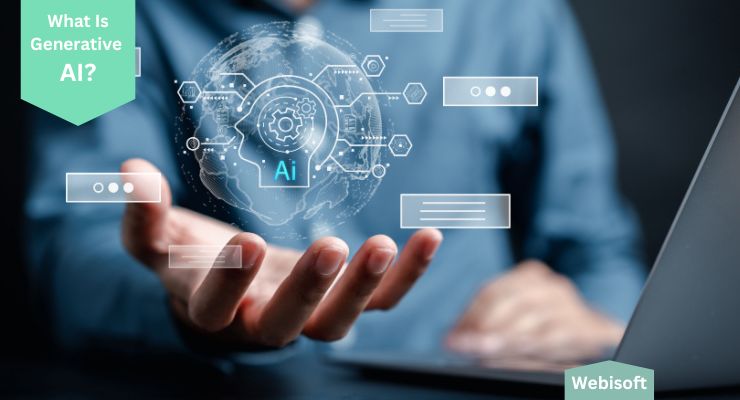
Generative AI is artificial intelligence’s subset that leverages machine learning techniques to generate data similar to the one it’s trained on.
It’s like giving an AI a creative mind, enabling it to produce new content, whether an image, a piece of music, or a block of text.
Generative AI is akin to a machine with a creative mind. It goes beyond data processing or making predictions. Generative AI creates, produces, and generates. The scope of its creativity is vast, spanning images, music, text, and intricate designs.
Machine learning techniques form the backbone of generative AI. These techniques enable the AI to learn from its training data. Over time, the AI grasps the patterns and structures in the data.
Once it understands these patterns, it can generate new data that follows the same patterns. Generative AI is not about replicating the training data. It’s about understanding the essence of the data and creating something new from it.
The generated data is not an exact copy of the training data. Instead, it’s a unique piece of data that shares the same characteristics as the training data. The power of generative AI lies in its versatility. It finds use in various applications.
In art, generative AI can create new artwork. In the music industry, it can compose new tunes. In content creation, it can write articles or generate social media posts.
Generative AI is making waves in the field of design. From creating new fashion designs to architectural blueprints, generative AI is a valuable tool. In gaming, generative AI can create new levels or characters.
Scientific and Medical Applications
In the scientific community, generative AI is being used for research purposes. It can generate new hypotheses or simulate data for experiments. It can aid in drug discovery by generating new molecular structures in the medical field.
Generative AI is a testament to the advancements in artificial intelligence. It’s not just about machines processing data or performing tasks. It’s about machines creating, innovating, and contributing to the world of creativity.
Generative AI offers a glimpse into the future of artificial intelligence, a future where machines can be creators.
A Brief History of Generative AI
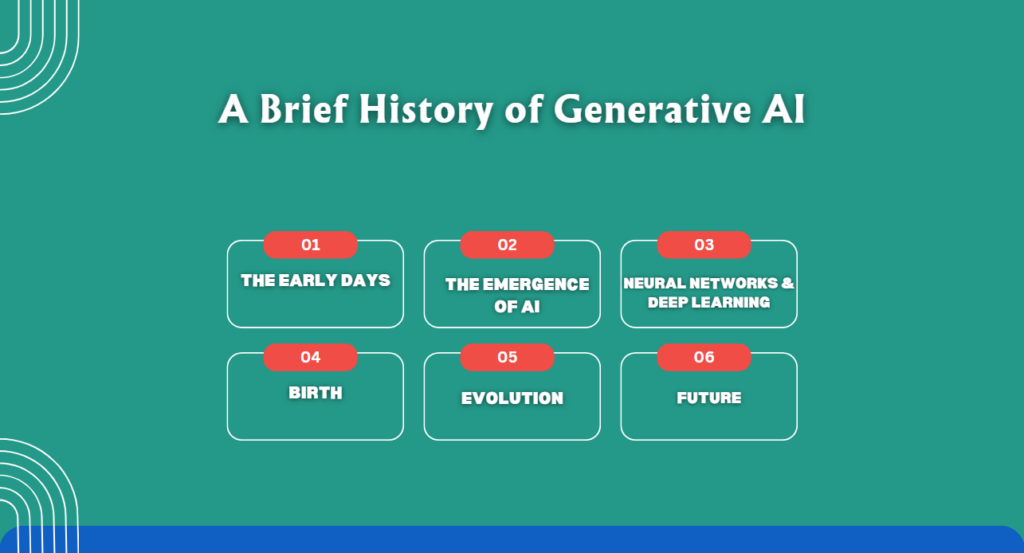
The concept of generative AI has been introduced previously. It dates back to the 1940s and 1950s, when the foundation for artificial intelligence was laid.
However, it wasn’t until the 2010s, with the advent of deep learning and neural networks, that generative AI took the shape we know today.
The journey of generative AI is a fascinating one. Its roots trace back to the mid-20th century, but its true potential has only been realized in the last decade.
1. The Early Days
Generative AI’s story begins in the 1940s and 1950s. During this period, the groundwork for artificial intelligence was established.
However, the concept of generative AI was still in its infancy. The technology and computational power required to realize it were yet to be available.
2. The Emergence of AI
As the decades passed, advancements in technology and computational power led to the emergence of artificial intelligence.
Machines began to learn from data, make predictions, and perform tasks. Yet, the concept of generative AI remained unexplored.
3. The Advent of Neural Networks & Deep Learning
The 2010s marked a turning point for generative AI. The advent of neural networks revolutionized artificial intelligence. These technologies allowed machines to learn from data in a way impossible before.
They could understand complex patterns and structures in the data, paving the way for the development of generative AI.
4. The Birth of Generative AI
With the beginning of deep learning and neural networks, generative AI started to take shape. Machines were not just learning from data; they were creating new data.
They were generating images, composing music, and writing text. The era of generative AI had begun.
5. The Evolution of Generative AI
Since its inception, generative AI has continued to evolve. New models and techniques have been developed, each more advanced than the last. Today, generative AI can create data almost indistinguishable from the data it’s trained on.
6. The Future of Generative AI
The future of generative AI is promising. With advancements in machine learning and computational power, generative AI will become more accurate, efficient, and versatile. It will continue to reshape industries and redefine creativity.
In conclusion, the history of generative AI is a testament to technological progress and human ingenuity. From its humble beginnings in the mid-20th century to its current state of the art, generative AI has come a long way.
The Generative AI Tech Stack
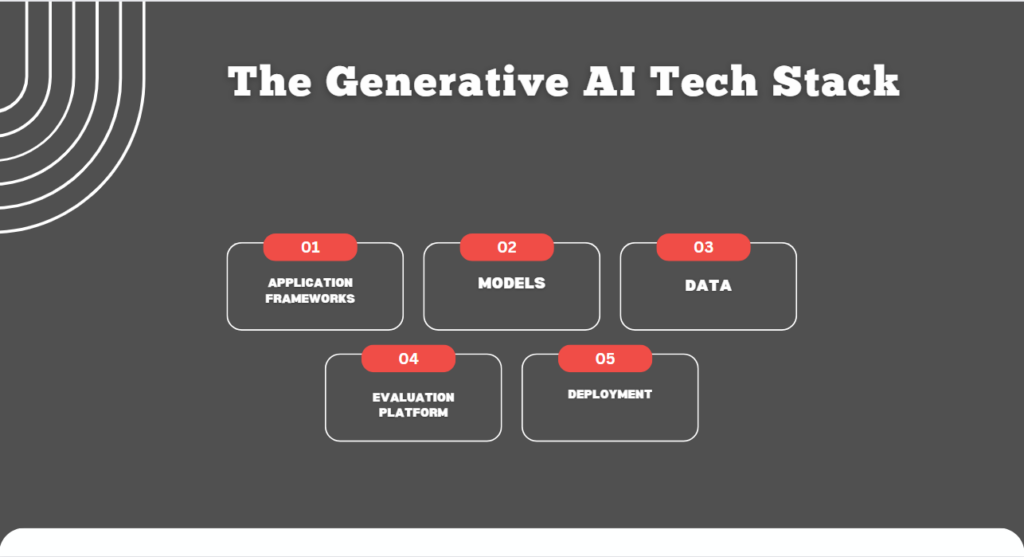
The tech stack for generative AI combines various components to create, evaluate, and deploy generative models.
The technology stack for generative AI is a complex blend of various components. Each element is crucial, working harmoniously to create, evaluate, and deploy generative models.
1. Application Frameworks
Application frameworks are the bedrock of generative AI. These are software libraries explicitly designed to support the development of machine learning applications.
They provide predefined functions and structures, significantly simplifying the process of creating complex models. Some of the most famous examples of these frameworks include TensorFlow, PyTorch, and Keras.
These frameworks serve as generative AI, providing the necessary tools and functionalities that make it easier for developers to create and manipulate generative AI models.
2. Models
At the heart of generative AI are the models. These algorithms have been trained on a specific dataset to generate new data. Several types of models are used in generative AI, each with its unique characteristics and use cases.
The most common examples include Generative Adversarial Networks (GANs), Variational Autoencoders (VAEs), and autoregressive models.
These models are the engines that drive the generation of new data, learning from the training data and using that knowledge to create new, unique data.
3. Data
Data is the lifeblood of generative AI. The quality and quantity of data used to train a model significantly impacts the model’s ability to generate high-quality outputs.
The more diverse and extensive the dataset, the more accurate and creative the outputs of the generative AI model will be. Therefore, data collection and preprocessing are critical steps in developing generative AI models.
It involves gathering relevant data, cleaning it to remove any errors or inconsistencies, and formatting it in a way the models can easily use.
4. Evaluation Platform
An evaluation platform is a crucial tool or set of tools used to assess the performance of a generative model. These platforms help identify a model’s strengths and weaknesses, thereby guiding further improvements.
They provide various metrics and visualizations, allowing developers to understand how well their models perform and where improvements are needed. This feedback loop is essential for refining the model and ensuring it can produce the desired outputs.
5. Deployment
Deployment is the final stage in the generative AI tech stack. It involves integrating the trained model into an application or a system where it can serve its purpose.
It could be generating new content, making predictions, or any other task the model has been trained to perform. Deployment can be a complex process involving model optimization, server configuration, and API development.
It’s about making the model accessible and usable in a real-world context. The generative AI tech stack is a complex but well-structured system. Each component is crucial in creating, evaluating, and deploying generative models.
Understanding this tech stack is essential for anyone looking to delve into the generative AI world, whether a seasoned AI developer or a curious beginner.
It provides a roadmap for creating generative AI models and offers insight into the processes and technologies that make generative AI possible.
Different Types of Generative AI Models
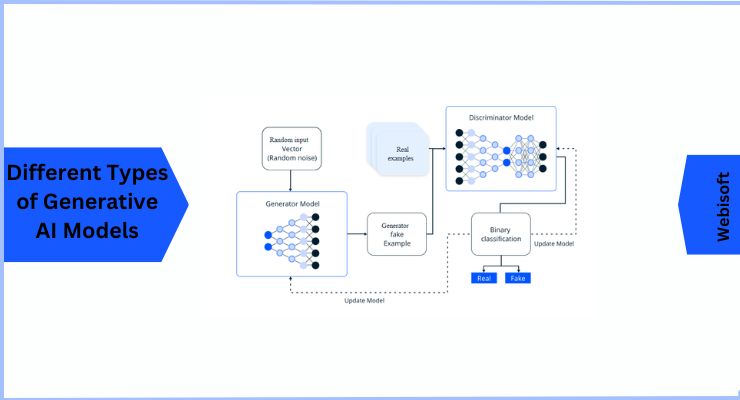
There are several generative models, each with unique characteristics and use cases. The world of generative AI is diverse, with several types of models. Each model has unique characteristics and use cases.
1. Generative Adversarial Networks (GANs)
GANs are a unique breed of generative models. They consist of two neural networks: a generator and a discriminator. The generator’s role is to create new data while the discriminator evaluates it.
The two networks work in tandem, improving each other in the process. The generator strives to create data that the discriminator can’t distinguish from accurate data.
Meanwhile, the discriminator continually enhances its ability to differentiate between accurate and generated data. This dynamic results in the generation of high-quality data.
2. Variational Autoencoders (VAEs)
VAEs add a layer of complexity to the traditional autoencoder model. They learn not just to compress data but also to generate new data.
This generation is achieved by sampling from the learned data distribution. VAEs are particularly useful when the goal is to generate new data that share the same underlying distribution as the training data.
3. Autoregressive Models
Autoregressive models predict the following output in a sequence based on previous outputs. They’re commonly used in time series forecasting and natural language processing.
For instance, in text generation, an autoregressive model can predict the next word in a sentence based on the words that came before it.
These models are powerful tools for generating sequences that follow the same patterns as the training data.
4. Flow-based Models
Flow-based models learn to transform data from a simple distribution to a complex one. This transformation enables them to generate new data that resembles the training data.
Flow-based models are particularly effective when the goal is to generate data in transformer-based model. It share the same complex distribution as the training data.
5. Rule-based Models
Rule-based models generate data based on a set of predefined rules. They are less flexible than other generative models but can be helpful in specific scenarios.
For instance, a rule-based model can ensure that all generated data adheres to these rules in a scenario where the data must follow a specific format or structure.
In conclusion, the landscape of generative AI models is diverse and dynamic. Each type of model offers unique capabilities, from GANs and VAEs to autoregressive and flow-based models.
Understanding these different models and their use cases is crucial for anyone looking to harness the power of generative AI.
How Does Generative AI Work?
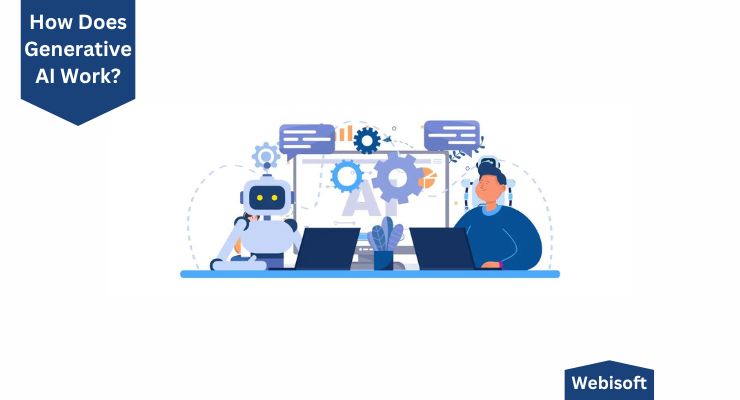
Generative AI learns the underlying patterns in the data it’s trained on. Once it understands these patterns, it can generate new data that follows the same patterns. This process involves complex algorithms and a lot of computational power.
The workings of generative AI are a blend of complex algorithms and computational power. It learns underlying patterns in the data it’s trained on and generates new data that follows these patterns.
Learning from Data
The first step in the process of generative AI is learning from data. The AI is trained on a specific dataset, a collection of images, a music library, or a text corpus.
During this training phase, the AI analyzes the data, looking for patterns and structures. For example, if the AI is trained on images of cats, it might learn about the shapes, colors, and textures that typically make up a cat image.
Understanding Patterns
Once the AI has been trained on the data, the next step is understanding the patterns in the data. It involves identifying the common characteristics and features in the training data.
Continuing with the cat image example, the AI might learn that cats typically have pointy ears, round eyes, and fur. This understanding of patterns is crucial for the next step – generating new data.
Generating New Data
After learning and understanding the patterns in the training data, the AI can start generating new data. This new data is created to follow the same patterns and structures that the AI learned from the training data.
In the cat image example, the AI would generate new images with the same features as a cat – pointy ears, round eyes, and fur. However, the generated images would not be exact copies of the training images.
Instead, they would be unique images with the same characteristics as the training images.
Complex Algorithms
Learning from data, understanding patterns, and generating new data involves complex algorithms. These algorithms are the backbone of generative AI, enabling it to perform its tasks.
Some of the most common algorithms used in generative AI include Generative Adversarial Networks (GANs), Variational Autoencoders (VAEs), and autoregressive models.
Computational Power
Generative AI needs a lot of computational power. Training the AI on the data, running the algorithms, and generating new data is computationally intensive.
It is why generative AI often requires high-performance hardware or cloud-based computing resources.
Continuous Improvement
One of the critical features of generative AI is its ability to improve continuously. As the AI generates new data, it can learn from the feedback it receives.
If the generated data is not high quality, the AI can adjust its algorithms and try again. This continuous cycle of generation, feedback, and adjustment allows the AI to improve over time, producing better and more accurate outputs.
In conclusion, generative AI is a complex and powerful technology. It works by learning from data, understanding patterns, and generating new data. This process, powered by complex algorithms and computational power.
As technologies continue developing and refining, we expect to see even more impressive results from generative AI.
Natural Language’s Role Processing in Generative AI
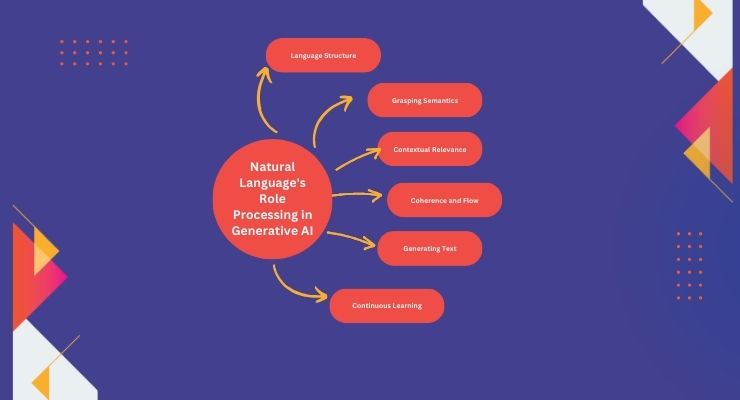
Natural language processing (NLP) is crucial in generative AI, especially when generating text. NLP techniques enable the AI to understand the structure and semantics of language, allowing it to produce coherent and contextually relevant sentences.
Natural Language Processing (NLP) holds a pivotal role in generative AI. It becomes exceptionally crucial when the task at hand involves generating text.
NLP techniques empower AI to comprehend the structure and semantics of language, enabling it to produce coherent and contextually relevant sentences.
1. Understanding Language Structure
One of the critical roles of NLP in generative AI is helping it understand language structure. Languages have complex structures with rules and patterns. NLP techniques enable AI to learn these structures.
For instance, a basic sentence structure in English might involve a subject, a verb, and an object. By learning such structures, AI can generate grammatically correct sentences.
2. Grasping Semantics
Beyond structure, language also involves semantics – the meaning of words and sentences. NLP plays a crucial role in helping AI understand semantics. Techniques such as word embedding allow AI to learn the meaning of words based on their context.
For example, the word “bank” could mean a financial institution or the side of a river, depending on the context. Understanding semantics allows AI to generate sentences that are not only grammatically correct but also make sense.
3. Contextual Relevance
Another important aspect of language is context. The sentence’s meaning can change dramatically based on the context in which it’s used.
NLP techniques help AI understand and consider the context when generating text. It ensures the generated text is correct, meaningful, and relevant to the context.
4. Coherence and Flow
In addition to structure, semantics, and context, NLP helps AI ensure coherence and flow in the generated text. This involves ensuring the sentences connect logically and the text flows smoothly.
Techniques such as sequence-to-sequence models can help, enabling AI to generate text that reads naturally, like a human wrote.
5. Generating Text
With an understanding of language structure, semantics, context, and coherence, AI can generate text.
It could be anything from a single sentence to a full-length article. The generated text quality depends on the quality of the training data and the sophistication of the NLP techniques used.
6. Continuous Learning
One of the advantages of using NLP in generative AI is the potential for continuous learning. The AI can learn and improve as it generates text and receives feedback.
It could involve refining its understanding of language structure and semantics, improving its ability to consider context, or enhancing the coherence and flow of the generated text.
NLP is crucial in generative AI, particularly text generation. It helps AI understand the complexities of language and generate grammatically correct, meaningful, contextually relevant, and coherent text.
As we continue developing and refining NLP techniques, we expect to see even more impressive results from generative AI in text generation.
Applications of Generative AI
Generative AI has a broad spectrum of applications across various industries. Its ability to generate new, unique data makes it a powerful tool in many fields.
Creative Industries
In the realm of creative industries, the application of generative AI serves as a powerful tool for content creation. It can generate new music, images, and text, providing artists unique ideas and inspirations.
For instance, AI can create music by learning the patterns in a dataset of songs and then generating new melodies that follow these patterns. Similarly, it can generate images or text that mimic the style of a given dataset.
Industrial Applications of Generative AI
Generative AI finds its applications in various industrial sectors as well. It can aid in designing new products by generating designs based on existing product datasets.
It can speed up the design process and lead to more innovative products. Additionally, generative Artificial intelligence can optimize processes by generating solutions to complex optimization problems.
It can also predict future trends by generating forecasts based on historical data. These applications can lead to significant improvements in efficiency and productivity in industries.
Medical Applications of Generative AI
The medical field also benefits from generative AI. It can assist in drug discovery by generating potential drug molecules based on a dataset of existing drugs.
Generative AI can also contribute to personalized medicine by generating treatment plans tailored to individual patients.
Furthermore, it can enhance medical imaging by generating high-quality images from low-quality inputs, aiding in more accurate diagnoses.
Financial Services
In the financial services sector, generative AI can be a powerful tool for risk assessment, fraud detection, and investment strategy development.
It can generate risk profiles based on a dataset of historical risk data, helping financial institutions make more informed decisions.
It can detect fraudulent transactions by generating patterns of regular transactions and comparing new transactions to these patterns. Additionally, it can develop investment strategies by generating predictions of market trends based on historical market data.
Chatbots and Voice Bots
Chatbots and voice bots powered by generative AI can provide more natural and engaging interactions. They can generate contextually relevant and linguistically correct responses, improving customer service and user experience.
The development of chatbots can be used in various sectors. It can be useful from customer service to personal assistants, providing users with more interactive and personalized experiences.
In conclusion, generative AI has various applications across various industries. Its ability to generate new, unique data makes it a powerful tool in many fields.
As we continue developing and refining generative AI technologies, we expect to see even more innovative applications.
Generative AI Use Cases
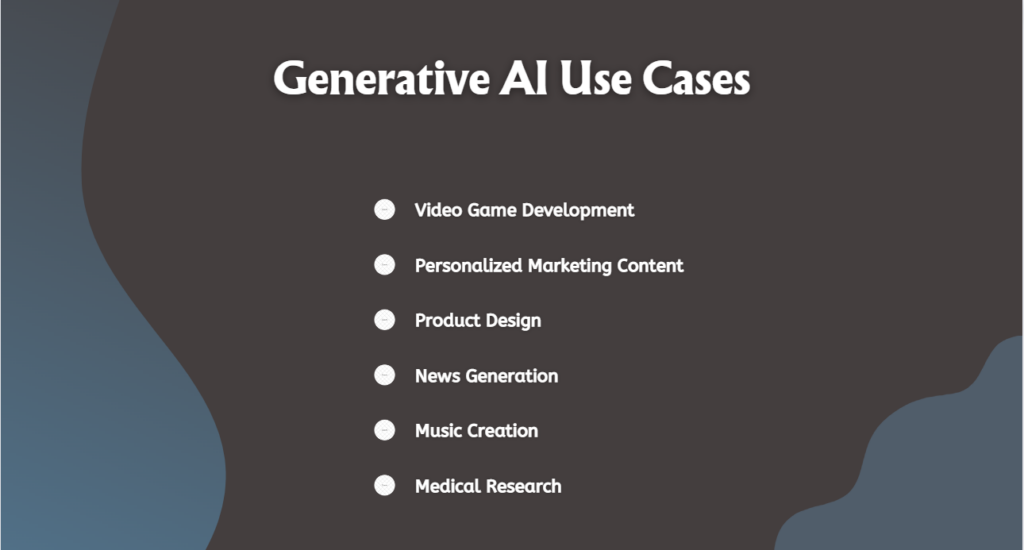
From creating realistic video game environments to generating personalized marketing content, the use cases for generative AI are vast and varied. We can expect to see even more innovative applications as the technology matures.
Generative AI has a plethora of use cases spanning various sectors. Its ability to create new, unique data opens up a world of possibilities.
1. Video Game Development
In video game development, generative AI can create realistic game environments. By learning the patterns in a dataset of real-world images, AI can generate new images that mimic these patterns.
This can result in more immersive and visually stunning game worlds, enhancing the gaming experience.
2. Personalized Marketing Content
Generative AI can also generate personalized marketing content. By learning from a dataset of customer profiles and past marketing campaigns, AI can generate new marketing content tailored to individual customers.
3. Product Design
In product design, generative AI can generate new design concepts. By learning from a dataset of existing designs, AI can generate new designs that follow the same patterns.
This can speed up the design process and lead to more innovative products.
4. News Generation
Generative AI can be used to generate news articles. By learning from a dataset of news articles, AI can generate new articles on a given topic. This can help news organizations produce content more quickly and efficiently.
5. Music Creation
In the field of music, generative AI can create new melodies. By learning the patterns in a dataset of songs, AI can generate new melodies that follow these patterns. This can lead to new musical compositions and new avenues for creativity.
6. Medical Research
In medical research, generative AI can generate potential drug molecules. By learning from a dataset of existing drugs, AI can generate new molecules that could be used as drugs.
In conclusion, the use cases for generative AI are vast and varied. From creating realistic video game environments to generating personalized marketing content, generative AI reshapes various sectors.
We can expect to see even more innovative applications as the technology matures. The potential of generative AI is vast, and we are just beginning to scratch the surface of what it can do.
The Future of Generative AI
The future of generative AI is promising. With advancements in machine learning and computational power, generative AI will become more accurate, efficient, and versatile. It will continue to reshape industries and redefine creativity.
The future of generative AI holds immense promise. As advancements in machine learning and computational power continue, generative AI is set to become more accurate, efficient, and versatile.
1. Increased Accuracy
In the future, we can expect generative AI to become more accurate. As machine learning algorithms become more sophisticated and training datasets become more extensive and more diverse, the quality of the data generated by AI will improve.
This means that the images, text, music, or any other data generated by AI will become more realistic and closely resemble the training data.
2. Improved Efficiency
Generative AI is also set to become more efficient. As computational power increases, AI can quickly process more significant amounts of data. This will reduce the time it takes to train AI models and generate new data.
Additionally, advancements in machine learning algorithms will improve efficiency by making the training process more effective.
3. Greater Versatility
The versatility of generative AI will also increase in the future. As AI becomes more sophisticated, it can generate a wider variety of data.
It could be used in broader applications, from creating realistic virtual reality environments to generating personalized medical treatments.
4. Industry Transformation
Generative AI will continue to reshape industries. AI will redefine creativity in the creative industries by providing artists with new tools and inspiration.
In industries like manufacturing and healthcare, AI will revolutionize processes by providing more efficient and effective solutions.
5. Ethical Considerations
As generative AI becomes more prevalent, ethical considerations will become increasingly important. Issues such as the potential misuse of AI-generated content and the impact of AI on jobs will need to be addressed.
Ensuring that ethical principles guide the development and use of generative AI will be crucial. The future of generative AI is promising.
With advancements in machine learning and computational power, generative AI will become more accurate, efficient, and versatile.
It will continue to reshape industries and redefine creativity. However, as we embrace the potential of generative AI, it will also be essential to consider and address the ethical implications.
End Note
Generative AI is a powerful tool that’s reshaping the world as we know it. The future of generative AI is here, and it’s full of possibilities.With Webisoft’s generative AI solutions, you can innovate like never before.
From art to engineering, the possibilities are endless. Are you ready to redefine what’s possible? Webisoft’s generative AI is here to take your projects to the next level.